23rd July 2020 #cli #dropbox #golang #toolbox #waterminttoolbox I started building my first Go program in early 2016. I introduced it once on the blog in late 2017. I’d like to introduce the differences from the time I introduced it, and also what I’ve tried and achieved with the Go language after about 4 years of practice, as a reminder. In this article, I’ll show you how I designed and implemented the language in the first part of this article.
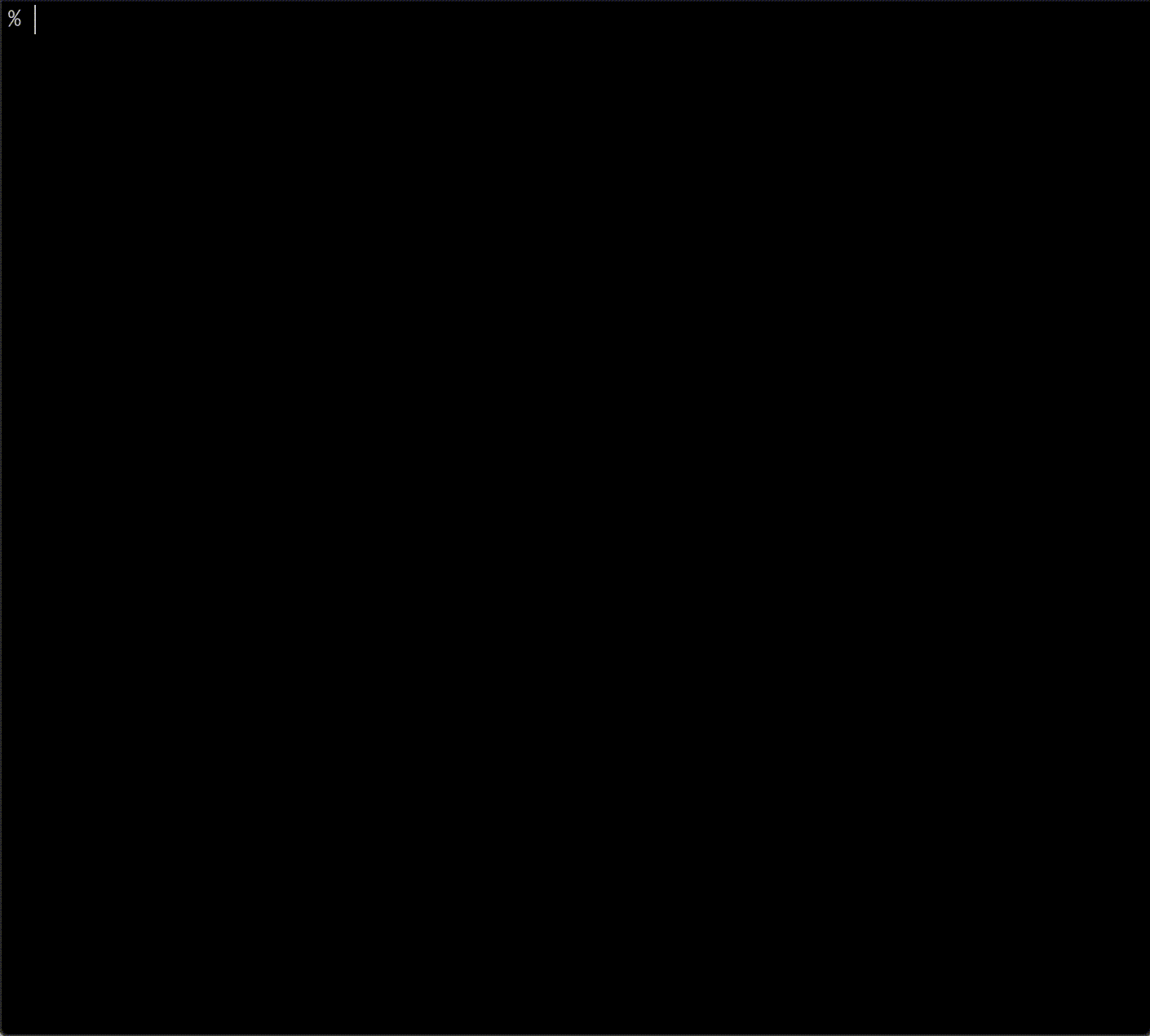
The project I spend the most time on in creating the Go program is a project called watermint toolbox. This is a tool that allows you to manage files, permissions, etc. in Dropbox and Dropbox Business from the command line. For example, if you want to list the group members on your team, you can run the following
watermint toolbox 71.4.504
==========================
© 2016-2020 Takayuki Okazaki
Licensed under open source licenses. Use the `license` command for more detail.
Testing network connection...
Done
Scanning: Developer Inc のメンバー全員
Scanning: Okinawa
Scanning: Osaka
Scanning: Tokyo
group_name group_management_type access_type email status surname given_name
xxxxxxxxx Inc のメンバー全員 system_managed member xxx.xxx@xxxxxxxxx.xxx active 杉戸 宏幸
xxxxxxxxx Inc のメンバー全員 system_managed member xxx.xxxx@xxxxxxxxx.xxx active 藤沢 由里子
xxxxxxxxx Inc のメンバー全員 system_managed member xxx.xxxx@xxxxxxxxx.xxx active 江川 紗和
xxxxxxxxx Inc のメンバー全員 system_managed member xxx.xxx@xxxxxxxxx.xxx active 正木 博史
xxxxxxxxx Inc のメンバー全員 system_managed member xxx.xxxxx@xxxxxxxxx.xxx invited
xxxxxxxxx Inc のメンバー全員 system_managed member xxx.xxx@xxxxxxxxx.xxx active Dropbox Debugger
xxxxxxxxx Inc のメンバー全員 system_managed member xxx.xxx@xxxxxxxxx.xxx active 里咲 広瀬
xxxxxxxxx Inc のメンバー全員 system_managed member xxx.xxx@xxxxxxxxx.xxx active 関本 重信
The report generated: /Users/xxxxxxxx/.toolbox/jobs/20200723-101557.001/report/group_member.csv
The report generated: /Users/xxxxxxxx/.toolbox/jobs/20200723-101557.001/report/group_member.json
The report generated: /Users/xxxxxxxx/.toolbox/jobs/20200723-101557.001/report/group_member.xlsx
(People’s names are pseudonyms, some output results have been replaced.)
The result report is output to standard output and the details are saved to a file in CSV, xlsx or JSON format. You can select Markdown or JSON as the output format for the standard output, but if you select JSON, you can easily extract the group name and email address from the above mentioned output and convert them to CSV by using the jq command.
% tbx group member list -output json | jq -r '[.group.group_name, .member.profile.email] | @csv'
"xxxxxxxxx xxx のメンバー全員","xxx+xxx@xxxxxxxxx.xxx"
"xxxxxxxxx xxx のメンバー全員","xxx+xxxx@xxxxxxxxx.xxx"
"xxxxxxxxx xxx のメンバー全員","xxx+xxxx@xxxxxxxxx.xxx"
"xxxxxxxxx xxx のメンバー全員","xxx+xxx@xxxxxxxxx.xxx"
"xxxxxxxxx xxx のメンバー全員","xxx+xxxxx@xxxxxxxxx.xxx"
"xxxxxxxxx xxx のメンバー全員","xxx+xxx@xxxxxxxxx.xxx"
"xxxxxxxxx xxx のメンバー全員","xxx+xxx@xxxxxxxxx.xxx"
"xxxxxxxxx xxx のメンバー全員","xxx+xxx@xxxxxxxxx.xxx"
(Some of the output results have been replaced.)
It also implements more than 100 other commands such as file upload, list of shared links, add and remove members, and more. Please refer to the README for more details on what you can do.
Requirements and constraints
The purpose of this tool development is to improve operational efficiency and solve issues in using Dropbox Business and Dropbox.
Dropbox has released a script that solves a common issue in the repository called DropboxBusinessScripts. On the other hand, this project is about being as easy to implement as possible to solve our goals, and in particular, not requiring additional libraries or configuration to use the tool, which was the main reason for choosing Go.
Since this program runs on a PC, its usage and messages should be easy to understand, and if a problem occurs, you should be able to find out the situation from a set of log files without having to ask for detailed information.
This project was a personal development project, there is mainly time resource constraints. There were times when I could develop a certain amount of code at a time, and other times when I didn’t write any code for several months. When I don’t write anything for a few months, the structure of the code disappears from my mind, so I had to suffer a lot of duplication and redoing.
The main requirements and constraints that I note from the above requirements and constraints are as follows. The reasons for each will be discussed below.
- distribution in a single binary (both executable and licensing point of view)
- Log every process to increase traceability
- Prioritize development productivity over CPU time
More recently, I have been paying attention to the following points as additional requirements
- Internationalization (Japanese and English)
- To save time, documents are basically auto-generated
- Do not overwhelm the disk with log files and intermediate files.
- Memory consumption should generally be kept to under a few hundred MB.
- Improved durability and optimized execution speed
I will recall how this project has been walked through with the above requirements and constraints in mind.
Selecting SDKs for APIs
At the time of writing, watermint toolbox has GitHub and Gmail related commands as well as Dropbox and Dropbox Business. These APIs are available as SDKs, so using these SDKs is more productive from the point of view of development productivity. I used to use SDKs in early stage of development, but I don’t use any SDKs including official and unofficial ones at this moment except OAuth2 implementation.
The SDK has both useful aspects and constraints. In particular, the constraints include the following
- In many cases, the log output cannot be changed in granularity or output method due to proprietary implementation.
- Error handling is blacked out
- There is a time lag between API updates and SDK updates.
Here’s a little more about each.
Log
This is not limited to the SDK, and it’s not limited to the Go language, but it’s quite difficult to unify the control to suppress the output or to get the trace log for debugging because each library logs to its own heart’s content. If you use the standard log library, you can switch the log output destination, but in the worst case, you can’t control the output by using fmt.Printf
. So you can send a pull request, give up, or create your own library. This time, I made the REST API framework from SDK for the reasons mentioned later, so I decided to make it by myself.
For log processing I used seelog in the beginning, but now I use zap wrapped in my own library. I think the main reason was that we wanted to change the JSON to a JSON that was easy to process in.
Instead of using zap as it is, I wrapped it in my own library for additional processing such as log rotation, compression, etc., and also for insurance in case I have to switch to a different log library at another time. As you know, it’s quite a hassle to switch log libraries.
Error handling is black boxed
In exception handling in languages with relatively strong types such as Java, classes are defined for each exception (e.g. AuthenticationException
for authentication errors, IOException
for network I/O problems, etc.) and the code that receives the exceptions handles them. You can determine and change the behavior.
You can define each error type in Go as well, but in some libraries, the entire process is rounded up into a string. This is probably more a cultural part of the library implementation than a language specification; some libraries throw everything as an Exception
or RuntimeException
in Java as well. However, as I have a long experience with Java, I feel that Go is more likely to suffer from this kind of problem.
For example, a case that still bothers me a bit is the golang/oauth2 Pull Request, errors: return all tokens fetch related errors as claimed in structured, where the errors are rounded to a string. Then it’s bit difficult to determine is the error retriable or not. Since this Pull Request seems to be neglected for more than a year, it’s quite difficult to decide whether to give up on Google’s implementation or to accept this as a specification and use it with a fixed version.
Other than this, I also experienced a black box error handling that made it look like a server error, even though it was a parameter error, and I wasted a lot of time trying to figure out what was going on. The accumulation of this wastage was a major driving force behind the plan to de-SDK.
Since the current watermint toolbox is implemented using my own REST API framework instead of using the SDK, all API requests and responses, except for those around OAuth2, are individually logged in JSON format, not to mention the trace log output during processing. For this reason, the reproducibility is high and it is possible to quickly determine whether the error is a parameter problem or a network problem.
Related commands have been added to improve productivity, for example, the API request and response of the last command executed can be output in JSON format. jq command makes it easy to extract the request with response code 409. Note that the token string is automatically replaced with for safety reasons.
% tbx job log last -kind capture -quiet | jq
{
"time": "2020-07-23T11:34:58.820+0900",
"msg": "",
"req": {
"method": "POST",
"url": "https://api.dropboxapi.com/2/team/get_info",
"headers": {
"Authorization": "Bearer <secret>",
"User-Agent": "watermint-toolbox/`dev`"
},
"content_length": 0
},
"res": {
"code": 200,
"proto": "HTTP/2.0",
"body": "{\"name\": \"xxxxxxxxx xxx\", \"team_id\": \"dbtid:xxxxxxxxxxxxxxxxxxxxxxxxxxxxxxxxxxx\", \"num_licensed_users\": 10, \"num_provisioned_users\": 8, \"policies\": {\"sharing\": {\"shared_folder_member_policy\": {\".tag\": \"anyone\"}, \"shared_folder_join_policy\": {\".tag\": \"from_anyone\"}, \"shared_link_create_policy\": {\".tag\": \"default_team_only\"}}, \"emm_state\": {\".tag\": \"disabled\"}, \"office_addin\": {\".tag\": \"disabled\"}}}",
"headers": {
"Cache-Control": "no-cache",
"Content-Type": "application/json",
"Date": "Thu, 23 Jul 2020 02:34:58 GMT",
"Pragma": "no-cache",
"Server": "nginx",
"Vary": "Accept-Encoding",
"X-Content-Type-Options": "nosniff",
"X-Dropbox-Request-Id": "xxxxxxxxxxxxxxxxxxxxxxxxxxxxxxxx",
"X-Envoy-Upstream-Service-Time": "77",
"X-Frame-Options": "SAMEORIGIN",
"X-Server-Response-Time": "71"
},
"content_length": 400
},
"latency": 384840087
}
(Some of the output results have been replaced.)
There is also a command that can be used to process this output and display the execution options with the curl
command. (Note: The latest release, 71.4.504 at the time of this writing, has a bug that prevents it from working. Please wait for release 72 or later.)
% tbx job log last -kind capture -quiet | tbx dev util curl
watermint toolbox `dev`
=======================
© 2016-2020 Takayuki Okazaki
オープンソースライセンスのもと配布されています. 詳細は`license`コマンドでご覧ください.
curl -D - -X POST https://api.dropboxapi.com/2/team/get_info \
--header "Authorization: Bearer <secret>" \
--header "User-Agent: watermint-toolbox/`dev`" \
--data ""
HTTP/2 200
cache-control: no-cache
content-type: application/json
date: Thu, 23 Jul 2020 02:34:58 GMT
pragma: no-cache
server: nginx
vary: Accept-Encoding
x-content-type-options: nosniff
x-dropbox-request-id: xxxxxxxxxxxxxxxxxxxxxxxxxxxxxxxx
x-envoy-upstream-service-time: 77
x-frame-options: SAMEORIGIN
x-server-response-time: 71
{"name": "xxxxxxxxx Inc", "team_id": "dbtid:xxxxxxxxxxxxxxxxxxxxxxxxxxxxxxxxxxx", "num_licensed_users": 10, "num_provisioned_users": 8, "policies": {"sharing": {"shared_folder_member_policy": {".tag": "anyone"}, "shared_folder_join_policy": {".tag": "from_anyone"}, "shared_link_create_policy": {".tag": "default_team_only"}}, "emm_state": {".tag": "disabled"}, "office_addin": {".tag": "disabled"}}}
(Some of the output results have been replaced.)
The API logs are currently also used for automated testing. I can replay the old API logs for various business logic to ensure that the business logic has not been affected by refactoring of the framework. I also have a command to anonymize log information for the preparation of data for testing, to improve efficiency.
There is a time lag between API updates and SDK updates.
When new APIs are added or parameters are added, it takes a certain amount of time for the SDK to keep up with those changes; in the case of the Dropbox API and the official SDK, the time lag is relatively short since the SDK is generated using the API’s DSL, called stone.
SDK for Go is positioned as unofficial, at the time of writing there are eight months’ worth of differences between the latest API and the one in use.
Whether or not to allow for this difference depends on your requirements, but in the watermint toolbox, I decided not to use the SDK because we wanted to try out the latest APIs easily.
One of the big advantages of using the SDK is that you don’t have to do the data structure definition yourself. As I’ve felt in the past when I was building tools to call REST APIs in Java and Scala, without using the SDK, most of the code was for defining data structures and mapping them to our own domain model. While the implementation work is monotonous and not very fun, I try to avoid this mapping work as much as possible because if I get it wrong, I get stuck in it. Again, in reinventing the REST API framework, I tried to keep the data structure definition to a minimum.
The following structure is designed to handle shared link data in the Dropbox API, but we decided to define only the minimum number of fields necessary to handle it in this tool.
type Metadata struct {
Raw json.RawMessage
Id string `path:"id" json:"id"`
Tag string `path:"\\.tag" json:"tag"`
Url string `path:"url" json:"url"`
Name string `path:"name" json:"name"`
Expires string `path:"expires" json:"expires"`
PathLower string `path:"path_lower" json:"path_lower"`
Visibility string `path:"link_permissions.resolved_visibility.\\.tag" json:"visibility"`
}
The first defined raw is the raw data returned by the API, and the following fields, such as Id and Tag, are automatically retrieved from path information in JSON, such as path:"id"
and path:"\\.tag"
.
Fields are defined in this way for items of high interest to users, such as shared link names, URLs, expiration dates, etc., that you want to output as reports, and other information is handled as JSON data only. Reports are output in three formats: CSV, xlsx, and JSON, but only these defined fields are output to CSV/xlsx. If you need information not included here, you can use the jq
command to extract it from the JSON output.
In order to easily retrieve only the information necessary for business purposes, it is sufficient to refer to CSV and xlsx format reports, and the data necessary for automation, such as id information, can be retrieved from the JSON format. By using these loose data structure definitions, the program can be used with almost no impact when fields are added to the API.
Addressing requirements and constraints
We will now look back again at how we have addressed the requirements and constraints mentioned above.
Single binary distribution
Being able to distribute a tool as a single binary is a very important theme for such a tool. If it is a tool that runs on my own PC or server, I can relax the library and OS requirements to some extent, but it is very important to support a wide range of OSs because it is a tool that runs on various users’ environments. The mechanism of single binary distribution is not limited to the Go language, but I think that the library group and the richness of the IDE have the advantage of being a certain pioneer in this field.
Although the watermint toolbox is licensed under the MIT license, it is linked with libraries under various other licenses. When selecting libraries, we mainly select the compatible BSD and Apache v2 licenses, and take care not to mix GPL licenses.
Log every process to increase traceability
Problems that occur in a user’s environment are often difficult to reproduce in my own environment, partly because of differences in the PC environment, and partly because of differences in the configuration, environment and size of the Dropbox or Dropbox Business where the API is used. For this reason, the logs are all output in the trace level. The log size is quite inflated, but I allow for the log size because priority to the time. Also, as described later, I’m currently using compression and log rotation to prevent the logs from overwhelming the disk.
Also, to make it easier to analyze the logs, the current version outputs all logs in JSON format (more precisely, JSON Lines format), to make it easier to extract errors and memory usage statistics by combining commands such as grep and jq
.
For example, by logging the last command executed and processing the results with jq
, you can get time by time heap usage statistics, as shown below. Such statistics can be useful in identifying the cause of the problem, since detailed data, such as memory profilers, are difficult to obtain in the user environment.
% tbx job log last -kind toolbox -quiet | jq -r 'select(.caller == "es_memory/stats.go:33") | [.time, .HeapAlloc] | @csv'
"2020-07-23T12:34:08.549+0900",104991184
"2020-07-23T12:34:13.548+0900",105058744
"2020-07-23T12:34:18.550+0900",111987752
Prioritize development productivity over CPU time
The watermint toolbox is a program that mainly makes API calls, processes the results and outputs them. The execution time ratio is relatively high in API processing wait time, and the ratio of CPU time is not high and can be almost negligible. Therefore, the programming style has changed in the last four years to be in line with it.
The biggest change is that I’ve been using Scala for a while now, and I’ve been trying to make my implementation functional and immutable. I’ve already failed to some extent in this endeavor, but I’ll talk about that later, but let’s start with the details.
I don’t remember where I read it, but I think it said that the idea of Go is that we should not hide the complexity of the process in functions and so on. For example, when I need to sum the fields of some structure, my experience is that I usually think of cutting them out as functions to make them easier to reuse and test. Well, it’s not that hard to do.
type File struct {
Name string
Size int
}
func TotalSize(files []File) (total int) {
for _, file := range files {
total += file.Size
}
return
}
This works and there is no problem at all, but the above claim is that when you call TotalSize(), that does not tell whether it’s O(N) or O(N2) processing, but the caller can’t understand how much processing order is hidden behind it, so you should inline this kind of simple processing I think the argument was something like this. I think it was a superstition in the early days of my introduction to Go. It may be a superstition that I made a mistake at the beginning of my introduction to Go.
type File struct {
Name string
Size int
}
func MyBizLogic() {
// ...
var folder1Total int
for _, file := range folder1Files {
folder1Total += file.Size
}
// ...
var folder2Total int
for _, file := range folder2Files {
folder2Total += file.Size
}
}
Naturally, the code would be less readable, and productivity was significantly reduced due to mistyping and lack of test coverage. If you really wanted to care about processing order, it would be much more effective to name your functions with Hungarian naming, like ONLOGNTotal()
or ON2Total()
. I was completely superstitious.
Now that I understand myself that this is a superstition (it took me a couple of years to do so…), it’s only natural that you’d want to do the same array and hash operations that you’ve always done in Scala or Ruby. It’s natural to want to do the same kind of array and hash operations that you normally do in Scala or Ruby.
I looked at some existing libraries and decided to use go-funk as a reference. go-funk
makes full use of reflection to improve readability and productivity. As you can see in the Performance section of the project introduction, they don’t prepare functions for each type like ContainsInt()
does.
I thought about using go-funk
, but I decided to make my own to practice the Go language. The result is a library that looks like this. For example, you can define arrays a
and b
, and then extract the common elements.
a := es_array.NewByInterface(1, 2, 3)
b := es_array.NewByInterface(2, 3, 4)
c := a.Intersection(b) // -> [2, 3]
I thought I could make a library that is somewhat immutable and functional, but as I mentioned above “This attempt has already failed to some extent”, I ran into a wall in the language specification. The problem is the type information.
var folder1Files, folder2Files []File
// ... Getting folder1Files and folder2Files
// Convert from array to array interface for use in the library
folder1List := es_array.NewByInterface(folder1Files...)
folder2List := es_array.NewByInterface(folder2Files...)
// Extract the files common to folder1 and folder2
commonFiles := folder1List.Intersection(folder2List)
Now, this works to a certain extent, but the problem is that each time we deal with the resulting elements, we need to cast them.
commonFiles.Each(func (v es_value.Value) {
f := v.(File)
// Processing the element
})
Well, I’d say it’s not a problem, but while using the library makes it more efficient to some extent, it also increases the chance of a type exception at runtime, which is easy to solve in languages with strong types or type variables like Java and Scala, but difficult to solve in languages with weak types like Go. I guess.
sort.Strings()
in the standard library, passing an array for each type will reduce type-related errors. On the other hand, this will only work if the number of elements in the array remains the same, and it won’t work if you want to extract common elements from the two arrays or join the two arrays as described above.
menu := []string{" Soba", "Udon", "Ramen" }
sort.Strings(menu) // The array size doesn't change before and after processing
For now, I’m thinking about using this library in my entire project while covering the problems with unit tests, but if I come up with another good idea, I’d love to incorporate it into my work.
Internationalization
The watermint toolbox has been used in many countries, including Japanese users. Initially, the toolbox was only available in English, but since last year, we have been able to provide a Japanese language version.
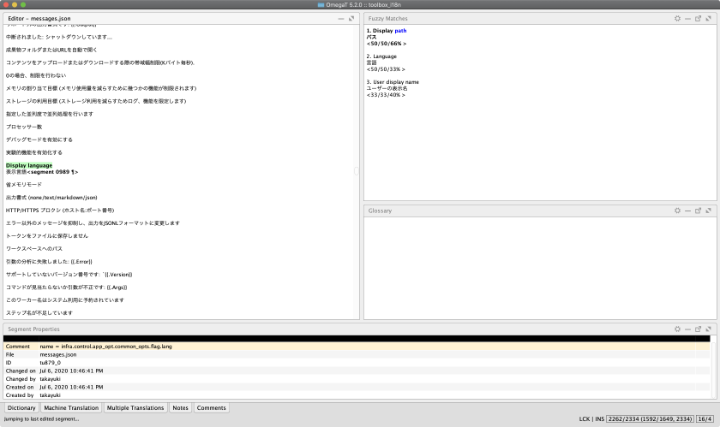
The translation memory software is OmegaT, with a plugin for the JSON data format.
There are a lot of libraries for internationalization, but I created them myself without thinking too much about it; I stored the message keys and translation text in JSON and switched the messages for display according to the language.
Now that I think about it, it may have been good to use go-i18n. go-i18n seems to use CLDR, and it seems to be able to handle differences of each language such as plural forms more finely. It may be worth trying to switch libraries at some point. Fortunately, I don’t think the switching cost is not so high because I don’t have deep implementation about internationalization.
To save time, documents are basically auto-generated
The most troublesome part of a personal project is the creation of the documentation. It takes a lot of time to create documents at the beginning, but it also takes some time to maintain them when the specifications change.
For this reason, the watermint toolbox automatically generates as much documentation as possible, including manuals. Although some explanations are missing, the ability to update the documentation in a comprehensive manner is a great advantage. It is not difficult to prepare manuals in the same format for multiple languages, as long as you have translated text.
However, automatic generation required several steps to be taken. Treating command specifications as programmatic data, extracting messages from the source code, detecting and reporting missing messages, generating update diffs for release notes, incorporating checks into the release process, and so on, I now have the ability to generate some readable manuals automatically.
The fact that command specifications can now be handled as data has been a nice side effect. Command specifications are stored in JSON format for each release, and this JSON makes it easier to extract, for example, a list of commands that take a CSV file as an argument.
% gzcat doc/generated/spec.json.gz | jq -r '.[] | select(.feeds | length > 0) | .path'
file dispatch local
file import batch url
group batch delete
member clear externalid
member delete
member detach
member invite
member quota update
member replication
member update email
member update externalid
member update profile
team activity batch user
team device unlink
team filerequest clone
teamfolder batch archive
teamfolder batch permdelete
teamfolder batch replication
In order to improve the efficiency of the debugging process, I spent a relatively large amount of time on the log output part. On the other hand, operations on large folders and teams took longer to execute and the log sizes were not negligible. Sometimes exceeding 100 GB. For this reason, recent releases incorporate a mechanism to gzip the logs and divide them into smaller chunks, deleting the old logs when they exceed a certain size.
Also, if it deletes the logs in order from the oldest, important data such as startup parameters may be lost, so I had to devise a way to output those data to a different log.
Implementing the log compression and rotation process itself was not too difficult, but I spent a lot of time trying to improve stability on Windows. This is due to deadlocks in a multi-threaded environment. There were several issues, including deadlocking of mutexes and waiting for I/O processing that still deadlocked. The frequency of reoccurrence was about once every hour to a few hours, so fixing and confirming the fix was quite a challenge.
I don’t think the code is durable enough for all conditions, but I believe the probable deadlock is mostly resolved. In addition, the deadlock was generally reproduced in the following code when connecting to the debugger.
type LogWriter struct {
w io.Writer
m sync.Mutex
}
func (z *LogWriter) Write(data []byte) (n int, err error) {
z.m.Lock()
defer z.m.Unlock()
return z.w.Write(data)
}
Indeed, it’s possible that Write()
itself could be deadlocked if it’s locked from the OS in some way. I haven’t researched the spec in detail, but that’s probably because I/O locking is more strict on Windows.
What you can see from the black box test is that when you select any text on a console, such as PowerShell, the scrolling locks and the standard output stops. On macOS and Linux, such a lock does not occur. I would like to investigate this issue in more detail if a similar issue occurs again.
Memory consumption should be generally kept to a few hundred MB.
If you use an external library, the memory consumption may be higher than expected. We have a solution for some of the libraries that use a lot of memory. I’ll introduce some concrete examples.
In addition to CSV and JSON, the watermint toolbox also provides the xlsx file format, which is used by Excel and others.
The CSV is enough for most cases, but if you try to read CSV with Japanese characters (UTF-8 encoding, no BOM) in Excel, Excel will not recognize the encoding correctly and the characters will be broken. You can add a BOM to the CSV side, but it will not be easy to use in programs that expect no-BOM. For this reason, I also support xlsx format output to avoid garbled characters in casual use.
Now the xlsx format is one of the Office Open XML, now standardized as ISO/IEC 29500. xlsx files are a collection of zipped files, and the files that contain the main data are made in the XML format.
Representing the spreadsheet in XML means that the entire spreadsheet needs to be expanded in memory once as a DOM or other XML tree. As the size of the spreadsheet grows, it uses proportionally more memory. Therefore, if you try to output the report in XML format, it will eat up the memory space proportionally according to the number of rows in the report. In some cases, this can cause an out of memory error and stop the process. As a countermeasure, the xlsx report is divided into separate files when the number of lines exceeds a certain number.
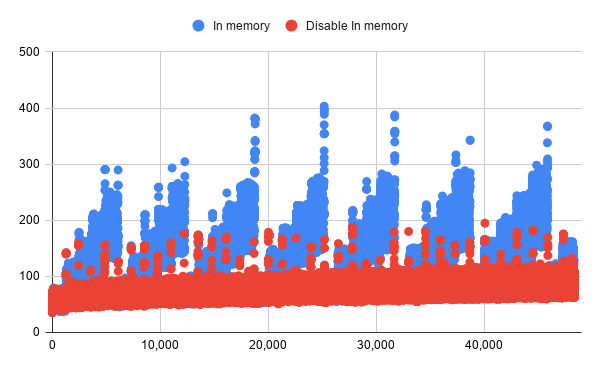
In addition to this, I also tuned the KVS, Badger. It use memory for caching, based on the number of data items. Again, the method of obtaining memory statistics from the log files was useful for long-term measurements with different parameters. The figure above shows the memory consumption trend for the two parameter settings.
Improved durability and optimized execution speed
Durability and execution speed are areas where there is still room for growth. In terms of fault tolerance, I have already implemented automatic retries when calling APIs, but I believe that there is room for improvement in error handling for the framework as a whole.
The watermint toolbox consists of commands, which are the core of the business logic, and the framework part that supports it. In most cases, the business logic part of the toolbox does not process errors returned by the API, and therefore, when an error occurs, it is returned to the upper framework.
func (z *List) Exec(c app_control.Control) error {
entries, err := sv_files.New(z.Peer.Context()).List(z.path)
if err != nil {
return err // return an error to the upper framework
}
// ... Subsequent processing
return nil
}
Network IO errors are handled by the REST API framework, and this is not a problem. But when retrieving a file list from a folder, is it necessary for the business logic that a different process is performed if the file does not exist, or is it an authentication error. I don’t know if this is an error or not, and I think it’s a bad idea to write the logic in various places.
Eventually I may settle again on the current form, but I may create a mechanism to explicitly separate what is handled by the framework from what is handled by the business logic, as there are attempts to implement try types, or to return on errors or to use panic()
.
There is still a lot of room for improvement in optimizing the execution speed. The current commands are programmed to be executed in multi-threads if they can be distributed to some extent, such as file uploads, folder permissions, etc. I don’t have to worry too much about it because the Go language has an environment for parallel processing such as goroutine and channels. I like the fact that it can be implemented.
On the other hand, if the execution time is longer than a few days, the overhead to reach the restart point becomes large, the intermediate file becomes bloated, and the progress is difficult to see. Therefore, it is time to consider introducing a processing framework that uses persistent asynchronous queues.
Summary
I have incorporated some of the development processes myself, such as the release process, as part of the tools, and our development efficiency is much better than it was four years ago. On the other hand, the Go language and the ecosystem surrounding it has allowed me to add unexpectedly advanced features to our specifications with ease. In the early days of development, Java, Scala, and Ruby were more experienced, so it was a bit of a struggle, but finally I’m starting to get the feeling that I can do most of the implementation in Go.
When I come up with an interesting implementation or a good library, I’d like to introduce it again.
22nd July 2020 #cli #dropbox #golang #toolbox #waterminttoolbox 初めてのGoプログラムを作り始めたのが2016年初旬。ブログでも2017年末に一度紹介しています。紹介した頃からの差分と、4年ほど実践してみたところでのGoという言語について試したこと、実現してきたことを備忘録がてら紹介します。紹介の前段としてどのような前提・要件をもとに設計・実装したか紹介していきます。
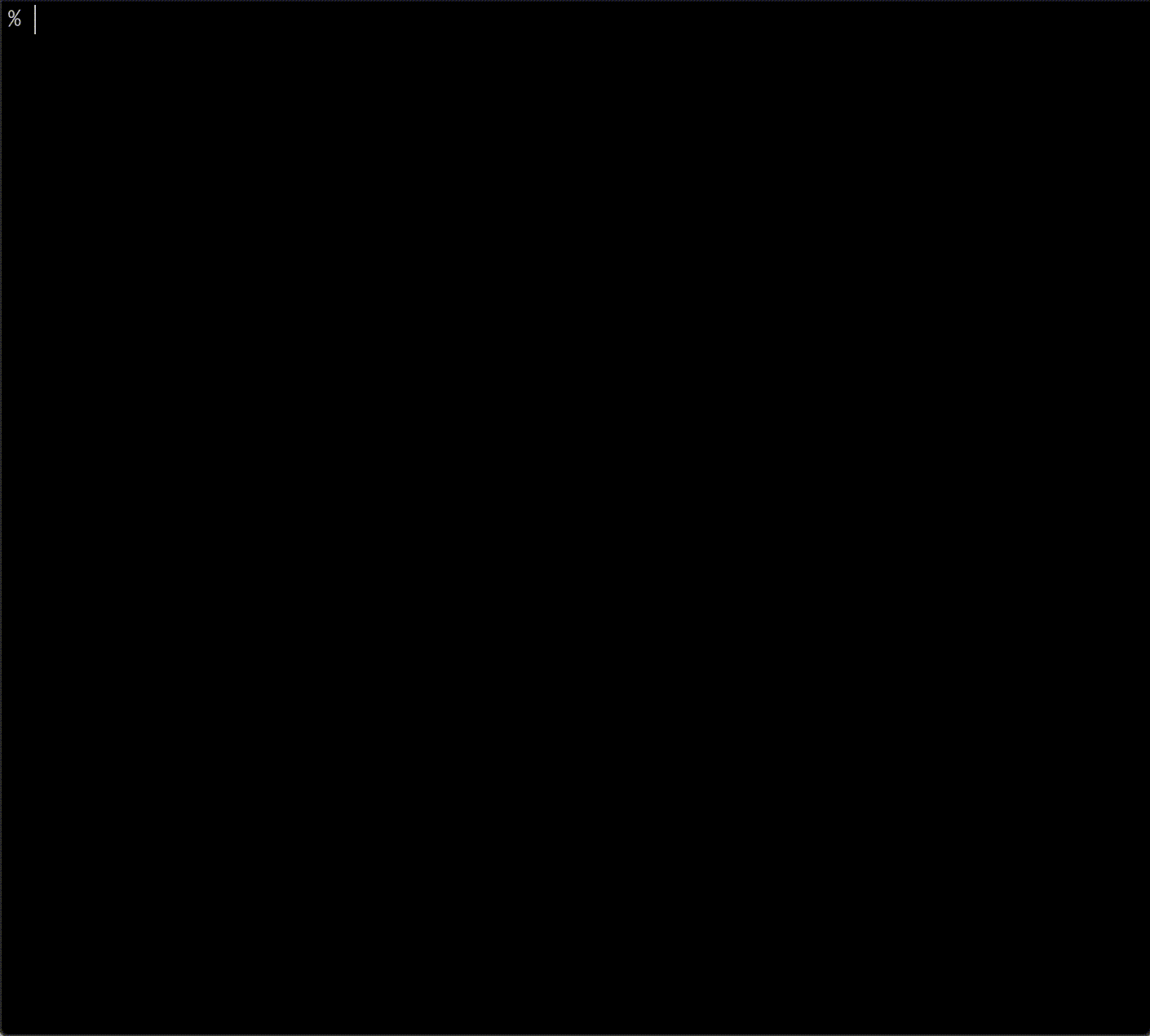
Goプログラムを作る中で最も時間をかけているのがwatermint toolboxというプロジェクトです。これは、コマンドラインからDropboxやDropbox Businessのファイルや権限などを管理するためのツールです。たとえば、チーム内のグループメンバーを一覧したい場合には次のように実行します。
$ ./tbx group member list
watermint toolbox 71.4.504
==========================
© 2016-2020 Takayuki Okazaki
オープンソースライセンスのもと配布されています. 詳細は`license`コマンドでご覧ください.
情報を取得中: Tokyo
情報を取得中: xxxxxxxxx Inc のメンバー全員
情報を取得中: Okinawa
情報を取得中: Osaka
group_name group_management_type access_type email status surname given_name
xxxxxxxxx Inc のメンバー全員 system_managed member xxx.xxx@xxxxxxxxx.xxx active 杉戸 宏幸
xxxxxxxxx Inc のメンバー全員 system_managed member xxx.xxxx@xxxxxxxxx.xxx active 藤沢 由里子
xxxxxxxxx Inc のメンバー全員 system_managed member xxx.xxxx@xxxxxxxxx.xxx active 江川 紗和
xxxxxxxxx Inc のメンバー全員 system_managed member xxx.xxx@xxxxxxxxx.xxx active 正木 博史
xxxxxxxxx Inc のメンバー全員 system_managed member xxx.xxxxx@xxxxxxxxx.xxx invited
xxxxxxxxx Inc のメンバー全員 system_managed member xxx.xxx@xxxxxxxxx.xxx active Dropbox Debugger
xxxxxxxxx Inc のメンバー全員 system_managed member xxx.xxx@xxxxxxxxx.xxx active 里咲 広瀬
xxxxxxxxx Inc のメンバー全員 system_managed member xxx.xxx@xxxxxxxxx.xxx active 関本 重信
レポートが作成されました: /Users/xxxxxxxx/.toolbox/jobs/20200723-101557.001/report/group_member.csv
レポートが作成されました: /Users/xxxxxxxx/.toolbox/jobs/20200723-101557.001/report/group_member.json
レポートが作成されました: /Users/xxxxxxxx/.toolbox/jobs/20200723-101557.001/report/group_member.xlsx
(人名は仮名です、一部出力結果は置換しています)
結果レポートは標準出力に出力されるほか、詳細はCSV、xlsx、JSON形式でファイルに保存されます。標準出力への出力形式はMarkdownやJSONも選択できます。JSONを選択すればjqコマンドなどと組み合わせて、前掲の出力からグループ名とメールアドレスだけを抽出してCSVに変換するといった操作も簡単です。
% tbx group member list -output json | jq -r '[.group.group_name, .member.profile.email] | @csv'
"xxxxxxxxx xxx のメンバー全員","xxx+xxx@xxxxxxxxx.xxx"
"xxxxxxxxx xxx のメンバー全員","xxx+xxxx@xxxxxxxxx.xxx"
"xxxxxxxxx xxx のメンバー全員","xxx+xxxx@xxxxxxxxx.xxx"
"xxxxxxxxx xxx のメンバー全員","xxx+xxx@xxxxxxxxx.xxx"
"xxxxxxxxx xxx のメンバー全員","xxx+xxxxx@xxxxxxxxx.xxx"
"xxxxxxxxx xxx のメンバー全員","xxx+xxx@xxxxxxxxx.xxx"
"xxxxxxxxx xxx のメンバー全員","xxx+xxx@xxxxxxxxx.xxx"
"xxxxxxxxx xxx のメンバー全員","xxx+xxx@xxxxxxxxx.xxx"
(一部出力結果は置換しています)
このほかにもファイルのアップロードや共有リンクの一覧、メンバーの追加・削除など100以上のコマンドを実装しています。どんなことができるか詳細はREADMEをご参照ください。
要件や制約
このツール開発ではDropbox BusinessやDropboxを利用する上での実運用での効率化や課題解決を目的としています。
DropboxからはDropboxBusinessScriptsというレポジトリでよく利用される課題を解消するスクリプトが公開されています。一方このプロジェクトでは、目的を解決するためになるべく簡単に実施できること、特にこのツールを使うにあたって追加のライブラリや環境設定を必要としないことをプロジェクトの意義としています。Goを選択した理由もまさにこの要件が最大の要因でした。
このプログラムはPC上で動作させますので、使い方やメッセージがわかりやすく、また不具合が発生した時には事細かに状況を聞かなくともログファイル一式から状況がわかるような仕組みも必要です。
このプロジェクトは個人的な開発で、主に時間的リソースの制約があります。ある程度まとめて開発できる時期もあれば、数ヶ月なにもコードを書かない時期もありました。数ヶ月何も書かないとコードの構成もさっぱりと頭から消えてしまうので、たくさんの重複実装とやり直し作業に苦しめられました。
以上の要件や制約から注意している主な要件・制約は次の通りになります。それぞれ理由は後述していきます。
- シングルバイナリで配布できること (動作上もライセンス上も)
- あらゆる処理をログに取得してトレーサビリティーを高める
- CPU時間よりも開発生産性を優先する
最近ではさらに追加要件として次のようなポイントにも気を配っています。
- 国際化対応 (日本語と英語)
- 時短のためにドキュメントは基本的に自動生成
- ログファイルや中間ファイルでディスクを圧迫しすぎないこと
- メモリの消費を概ね数百MB程度に抑えること
- 耐障害性の向上と、実行速度の最適化
以上の要件・制約を前提としてどのようにこのプロジェクトが歩んできたかを思い出していきます。
APIとSDKの選定
watermint toolboxでは執筆時点でDropbox・Dropbox Business以外にもGitHubとGmail関連コマンドが実装されています。これらのAPIについてはそれぞれSDKが提供されていますのでこれを使うのが開発生産性の観点では有利です。開発初期にはSDKを使って開発をしていましたが、現時点ではOAuth2実装を除き公式・非公式含むSDKを利用していません。
SDKは便利な側面と、制約事項を併せ持っています。とくに制約事項には次のようなものがあります。
- ログ出力が独自実装で粒度や出力方法を変更できない場合が多い
- エラー処理がブラックボックス化されている
- APIの更新とSDKの更新では時差がある
それぞれ少し詳しく紹介します。
ログ出力が独自実装で粒度や出力方法を変更できない場合が多い
これはSDKだけに限りませんし、Go言語だけに限った話ではありませんが各ライブラリが思い思いにログ出力するため出力抑制あるいはデバッグのためのトレースログ取得といった制御の統一はかなり難しいところがあります。標準ログライブラリを使っていれば出力先を切り替えるなどできますが、酷い場合にはfmt.Printf
でログ出力しているなど制御が効かないケースもあります。このため地道にPull requestを送るか、諦めるか、あるいは自分でライブラリを作るかどれかの選択肢になります。今回は後述の理由などからSDKから自身でREST APIフレームワークを作りましたので、自身で作ることにしました。
ログ処理については最初の頃seelogを使っていましたが、いまではzapをさらに自身のライブラリでラップしたものを使っています。seelogからの乗り換え理由はあまり記憶が定かではありませんが、seelogでは設定のためにXMLが必要であったり、出力形式をjqで処理しやすいJSONに変更したかったのが大きな理由だったと思います。
zapをそのまま使うのではなく、わざわざさらに自身のライブラリでラップしたのはログローテーション、圧縮など追加処理のためと、またもし別の機会に違うログライブラリに乗り換えるケースが生じた場合への保険のためです。ご承知の通りログライブラリの入れ替えはかなり面倒ですから。。
エラー処理がブラックボックス化されている
Javaのように比較的強い型をもつ言語の例外処理では認証エラーならたとえばAuthenticationException、ネットワークI/O処理の問題であればIOExceptionといったように例外ごとにクラスが定義されそれぞれ例外を受け取ったコードが処理を判定し、振る舞いを変更できます。
Goでも同様にエラー種別ごとに定義はできますが、ライブラリによっては全ての処理を文字列として丸め込んでしまうケースがあります。これは言語仕様というよりはライブラリ実装上の文化的な部分が大きいでしょう。Javaでも同じようになんでもExceptionやRuntimeExceptionとしてスローするようなライブラリもあります。ただ、この辺の当たり外れというと失礼ですが苦しめられるケースはJava経験がそれなりに長い身としてはGoのほうが多いように感じます。
たとえば、今も少し悩んでいるケースとしてはgolang/oauth2のPull Request、errors: return all token fetch related errors as structuredで主張されている通り、エラーが文字列に丸められ、エラーがリトライ可能なものかどうか呼び出し側で判断できないというものがあります。1年以上このPull Requestも放置されているようなので、ここでGoogleの実装に見切りをつけるか、ある意味これを仕様として受け止めバージョンを固定した上で利用するかはかなり悩ましい部分です。
これ以外にも、エラー処理がブラックボックス化されていることによって、パラメータの間違いなのにサーバエラーのように見えてしまって原因究明にかなりの時間を浪費した経験もあります。この浪費の蓄積は脱SDKを計画する大きな原動力となりました。
現在のwatermint toolboxではSDKを使わず独自のREST APIフレームワークを使って実装しているので、処理中のトレースログ出力はもちろんのこと、OAuth2周りをのぞくすべてのAPIリクエスト・レスポンスを個別にJSON形式のログに残しています。このため、再現性も高くエラーがパラメータの問題なのか、ネットワークの問題なのかすぐに判定できるようになりました。
これに関連するコマンドも生産性向上のために追加してありたとえば、次のように最後に実行したコマンドのAPIリクエスト・レスポンスをJSON形式で出力できます。jqコマンドを使えばレスポンスコード409のリクエストだけ抽出するといった操作も簡単です。なお、トークン文字列は安全のために自動的に <secret>
と置換されます。
% tbx job log last -kind capture -quiet | jq
{
"time": "2020-07-23T11:34:58.820+0900",
"msg": "",
"req": {
"method": "POST",
"url": "https://api.dropboxapi.com/2/team/get_info",
"headers": {
"Authorization": "Bearer <secret>",
"User-Agent": "watermint-toolbox/`dev`"
},
"content_length": 0
},
"res": {
"code": 200,
"proto": "HTTP/2.0",
"body": "{\"name\": \"xxxxxxxxx xxx\", \"team_id\": \"dbtid:xxxxxxxxxxxxxxxxxxxxxxxxxxxxxxxxxxx\", \"num_licensed_users\": 10, \"num_provisioned_users\": 8, \"policies\": {\"sharing\": {\"shared_folder_member_policy\": {\".tag\": \"anyone\"}, \"shared_folder_join_policy\": {\".tag\": \"from_anyone\"}, \"shared_link_create_policy\": {\".tag\": \"default_team_only\"}}, \"emm_state\": {\".tag\": \"disabled\"}, \"office_addin\": {\".tag\": \"disabled\"}}}",
"headers": {
"Cache-Control": "no-cache",
"Content-Type": "application/json",
"Date": "Thu, 23 Jul 2020 02:34:58 GMT",
"Pragma": "no-cache",
"Server": "nginx",
"Vary": "Accept-Encoding",
"X-Content-Type-Options": "nosniff",
"X-Dropbox-Request-Id": "xxxxxxxxxxxxxxxxxxxxxxxxxxxxxxxx",
"X-Envoy-Upstream-Service-Time": "77",
"X-Frame-Options": "SAMEORIGIN",
"X-Server-Response-Time": "71"
},
"content_length": 400
},
"latency": 384840087
}
(一部出力結果は置換しています)
また、この出力を加工して curl
コマンドで実行オプションを表示するコマンドもあります。(注: 執筆時点の最新リリース71.4.504にはバグがありうまく動作しません。リリース72以降をお待ちください)
% tbx job log last -kind capture -quiet | tbx dev util curl
watermint toolbox `dev`
=======================
© 2016-2020 Takayuki Okazaki
オープンソースライセンスのもと配布されています. 詳細は`license`コマンドでご覧ください.
curl -D - -X POST https://api.dropboxapi.com/2/team/get_info \
--header "Authorization: Bearer <secret>" \
--header "User-Agent: watermint-toolbox/`dev`" \
--data ""
HTTP/2 200
cache-control: no-cache
content-type: application/json
date: Thu, 23 Jul 2020 02:34:58 GMT
pragma: no-cache
server: nginx
vary: Accept-Encoding
x-content-type-options: nosniff
x-dropbox-request-id: xxxxxxxxxxxxxxxxxxxxxxxxxxxxxxxx
x-envoy-upstream-service-time: 77
x-frame-options: SAMEORIGIN
x-server-response-time: 71
{"name": "xxxxxxxxx Inc", "team_id": "dbtid:xxxxxxxxxxxxxxxxxxxxxxxxxxxxxxxxxxx", "num_licensed_users": 10, "num_provisioned_users": 8, "policies": {"sharing": {"shared_folder_member_policy": {".tag": "anyone"}, "shared_folder_join_policy": {".tag": "from_anyone"}, "shared_link_create_policy": {".tag": "default_team_only"}}, "emm_state": {".tag": "disabled"}, "office_addin": {".tag": "disabled"}}}
(一部出力結果は置換しています)
このAPIログは現在ほかに自動テストのためにも利用しています。各種ビジネスロジックに対して過去のAPIログをリプレイテストすることによりフレームワークのリファクタリングなどによりビジネスロジックが影響を受けていないことを確認できます。また、テスト用のデータ準備のためにログ情報を匿名化するためのコマンドも準備して効率化を図っています。
APIの更新とSDKの更新では時差がある
新しいAPIが追加されたり、パラメータが追加された場合にSDK側がそれらの変更に追従するには一定の時間がかかります。Dropbox APIと公式SDKの場合、stoneというAPI定義のDSLを使ってSDKを生成しているのでタイムラグは比較的短いですがGo向けのSDKは非公式という位置付けのためか執筆時点で最新APIと8ヶ月分の差分があります。
この差分を許容するかどうかは要件次第ですが、watermint toolboxでは最新APIを気軽に試してみたいという気持ちが強くありSDKを使わない方向へと流れを決めていきました。
SDKを使う大きなメリットの一つとしてデータ構造定義を自分で行わなくて良い点があります。以前JavaやScalaを使ってREST APIを呼び出すツールを作っていたときからも感じていましたが、SDKを使わない場合、ほとんどのコードはデータ構造定義と自前のドメインモデルとのマッピングのためのものでした。実装作業も単調であまり楽しくない割に、間違えるととことんはまるのでこのマッピングのための作業はなるべく避けたいと考えています。今回、またREST APIフレームワークを再発明するにあたっては、データ構造定義は最低限で済むように心がけました。
次の構造体はDropbox APIの共有リンクデータを取り扱うためのものですが、このツールでは必要最小限のフィールドのみを定義して対応することにしました。
type Metadata struct {
Raw json.RawMessage
Id string `path:"id" json:"id"`
Tag string `path:"\\.tag" json:"tag"`
Url string `path:"url" json:"url"`
Name string `path:"name" json:"name"`
Expires string `path:"expires" json:"expires"`
PathLower string `path:"path_lower" json:"path_lower"`
Visibility string `path:"link_permissions.resolved_visibility.\\.tag" json:"visibility"`
}
一番最初に定義されているRaw
はAPIから返された生データで、続くId
やTag
などのフィールドは path:"id"
や path:"\\.tag"
といったJSON上のパスを示す付加情報から自動取得するようになっています。
共有リンクの名前、URL、有効期限などレポートとして出力したい、ユーザーの関心が高い項目はこのようにフィールドを定義し、それ以外の情報はJSONデータのみとして取り扱っています。レポートはCSV、xlsx、JSON形式の3種類で出力されますが、CSV・xlsxにはこの定義されたフィールドのみが出力されます。ここに含まれない情報が必要な場合にはJSON出力からjq
コマンドなどで取り出すという流れになります。
ビジネス上必要な情報のみを気軽に取り出すためにはCSV・xlsx形式レポートを参照すればよく、Id情報など自動化のためにプログラム上必要なデータはJSON形式から取り出せるという使い分けです。このようなゆるいデータ構造定義を使うことにより、API使用上フィールドが追加された場合でも影響をほとんど受けることなくプログラムを使えるようになりました。
要件や制約事項への対応
それではまた前掲の要件や制約事項についてどのような対応をしたか振り返っていきます。
シングルバイナリで配布できること
シングルバイナリで配布できることはこのようなツールにとってとても大きなテーマです。自社内のPCやサーバーで実行するツールであればある程度ライブラリ・OS要件を緩和しても良いのですが、様々なユーザーの環境で動作するツールのため対応OSの幅はとても重要です。シングルバイナリで配布する仕組みはGo言語に限ったものではありませんが、ライブラリ群やIDEの充実度はこの分野のあるいみパイオニアならではの強みがあると考えています。
またコンパイル済みバイナリとして提供するにあたってはライセンス形態にも気を配る必要があります。watermint toolbox本体はMITライセンスですが、ほかにも様々なライセンスのライブラリをリンクしています。ライブラリを選定するにあたっては相性の良いBSD、Apache v2ライセンスを中心に選定し、GPLは混在しないように気を付けています。
あらゆる処理をログに取得してトレーサビリティーを高める
ユーザーの環境で発生する問題は多くの場合、手元の環境で再現が難しいものです。PC環境の違いもありますし、APIを使う先のDropboxやDropbox Businessの設定・環境・規模が違うケースがあるためです。このため、ログはトレースレベルでのログを全て出力しています。ログサイズはかなり膨れ上がりますが、デバッグのための時間的制約がより優先度が高いためログサイズについては許容しています。また、後述の通りログでディスクを圧迫しないよう現在は圧縮・ログローテーションを実施しています。
またログを解析しやすいよう現バージョンではすべてJSON形式(正確にはJSON Lines形式)でログ出力しています。grepやjqといったコマンドを組み合わせることでエラーやメモリ利用統計などを抽出しやすくするためです。
たとえば、次のように最後に実行したコマンドのログを取得し、その結果をjqで加工すれば時刻ごとのヒープの利用統計を取得できます。ユーザー環境ではメモリプロファイラなど詳細なデータ取得が難しいため、このような統計取得は原因の特定に役立ちます。
% tbx job log last -kind toolbox -quiet | jq -r 'select(.caller == "es_memory/stats.go:33") | [.time, .HeapAlloc] | @csv'
"2020-07-23T12:34:08.549+0900",104991184
"2020-07-23T12:34:13.548+0900",105058744
"2020-07-23T12:34:18.550+0900",111987752
CPU時間よりも開発生産性を優先する
watermint toolboxは主にAPI呼び出しし、その結果を加工して出力するだけのプログラムです。実行時間は相対的にAPI処理待ち時間が多く、CPU時間の比率は高くなくほとんど無視できます。このため、プログラミングスタイルもそれに準じた格好にこの4年で変化してきました。
一番おおきな変化はScalaを一時期使っていたこともあり関数型・イミュータブルな実装を少しずつ目指そうとしているところです。この試みはすでにある程度失敗しているのですが、失敗談は後述するとしてまず細かな考え方の変遷から。
どこで読んだのかは失念しましたが、Goの考え方として関数などに処理の複雑性を隠さないようにすべきと書かれていたと思います。たとえば、何かの構造体のフィールドの合計を取る処理が必要なとき、今までの経験では普通にそれらを再利用・テストしやすいよう関数として切り出すことを考えます。まあ、そんなに難しいことではありません。
type File struct {
Name string
Size int
}
func TotalSize(files []File) (total int) {
for _, file := range files {
total += file.Size
}
return
}
これはこれで動作しますし全く問題ないのですが、前掲の主張ではTotalSize()
を呼び出す際O(N)の処理なのか、O(N^2)の処理なのかわからないがどのぐらいの処理オーダーが背後に隠れているか呼び出し側では区別できないからこういった簡単な処理はインライン化すべきである。といったような主張だったとおもいます。もはやGo入門初期に間違えてみた迷信だったのかもしれません。
type File struct {
Name string
Size int
}
func MyBizLogic() {
// ...
var folder1Total int
for _, file := range folder1Files {
folder1Total += file.Size
}
// ...
var folder2Total int
for _, file := range folder2Files {
folder2Total += file.Size
}
}
当然ながらコードの可読性が下がりますし、ミスタイプやテストカバレッジ不足で生産性は著しく低下しました。もし本当に処理オーダーを気にしたいのであれば、関数名にハンガリアンネーミングでONLOGNTotal()
とかON2Total()
のように命名した方がよほど効果的でしょう。完全に迷信でした。
さて、この考え方が迷信であることが自分の中で確定したところで(確定するまでに2年ぐらいかかりました…)、ScalaやRubyなどいつもやっているような配列、ハッシュ操作を同じようにやりたいという欲求にかられるのは自然なことです。配列Aと配列Bに共通する要素だけ抽出したり、前掲の合計値のような処理もプログラムの性質上それなりに多く扱いますのでこういった処理の効率化は大きな改善につながります。
いくつか既存ライブラリを調べましたがgo-funkというプロジェクトが興味深く参考にしていくことにしました。go-funkではリフレクションをフル活用して可読性・生産性向上を目在しています。プロジェクト紹介のPerformanceにもある通り、ContainsInt()
のように型ごとに関数を用意したりはしないという方向性からもその目標がよくわかります。
go-funkを使おうかと思いましたが、Go言語の練習のためということで自作することにしました。出来上がったのは次のようなライブラリです。 たとえば配列をa
、b
と定義して、共通する要素を取り出すというシンプルな関数が実装が出来上がりました。
a := es_array.NewByInterface(1, 2, 3)
b := es_array.NewByInterface(2, 3, 4)
c := a.Intersection(b) // -> [2, 3]
これである程度イミュータブルかつ関数型的にかけるライブラリが作れるかと思ったのですが、前述の「この試みはすでにある程度失敗」との通り言語仕様上の壁にぶつかりました。問題は型情報です。
var folder1Files, folder2Files []File
// ... folder1Files, folder2Files の取得
// 配列からライブラリで使う配列インタフェースに変換
folder1List := es_array.NewByInterface(folder1Files...)
folder2List := es_array.NewByInterface(folder2Files...)
// folder1, folder2に共通するファイルを抽出
commonFiles := folder1List.Intersection(folder2List)
さてここまではある程度動作するのですが、問題はこの結果得られる要素それぞれを扱うときに毎回キャストが必要となるところです。
commonFiles.Each(func (v es_value.Value) {
f := v.(File)
// 要素に対する処理
})
まあ問題ないと言えば問題ないのですが、ライブラリを使うことである程度効率化できる一方実行時の型例外が発生する可能性が増えてしまいました。JavaやScalaなど強い型あるいは型変数がある言語での解消は簡単ですが、Goのように弱い型の言語ではこの解決は難しいでしょう。
標準ライブラリのsort.Strings()
のように型ごとの配列を渡して処理する方式にすると型に関するエラーは減少します。一方で、これが通用するのは配列の要素数が変わらない時だけで前掲の2つの配列から共通要素を取り出したり、配列を結合したりするケースには使えません。
menu := []string{"そば", "うどん", "ラーメン"}
sort.Strings(menu) // 配列サイズは処理前後で変わらない
今のところは単体テストで問題をカバーしつつこのライブラリを全面的に使っていく方向で考えていますが、またいいアイデアがあればぜひ取り込んでいきたいと思っています。
国際化対応 (日本語と英語)
watermint toolboxは海外ユーザーにも多く使っていただいていますが、日本のユーザーにも使っていただいています。当初は英語のみ対応していましたが、昨年より日本語にも対応しました。
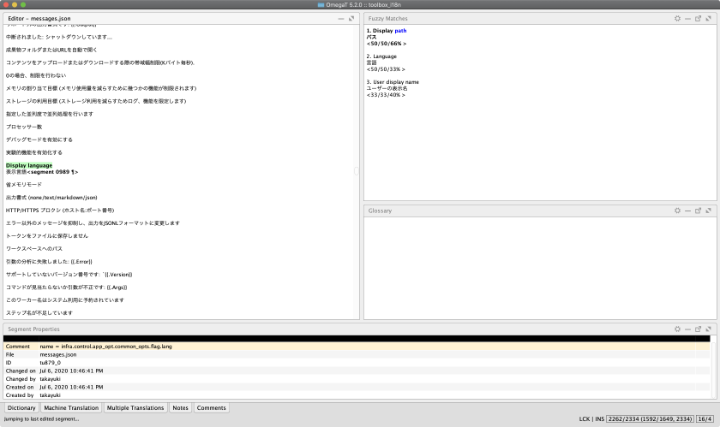
翻訳メモリソフトウエアはOmegaTを使っています。OmegaTにJSONデータ形式対応プラグインを導入して利用しています。
国際化のためのライブラリもたくさんありますが、あまり深く考えずに自作しています。JSONにメッセージのキーと翻訳テキストを格納し、表示用メッセージを言語によって切り替えています。
今思えばgo-i18nあたりを使っても良かったかもしれません。go-i18nではCLDRを使っているようで複数形など言語ごとの差分をより細かく吸収できるようです。いずれライブラリを切り替えるなど試してみる価値はありそうです。幸い、国際化については現状あまり深い実装をしていないため切り替えコストはさほど高くないと考えています。
時短のためにドキュメントは基本的に自動生成
個人プロジェクトとして比較的負担に感じる部分は一番面倒な部分はドキュメントの作成です。最初のドキュメント作成も手間がかかりますが仕様変更の際にドキュメント更新などメンテナンスもそれなりに時間がかかります。
このため、watermint toolboxではマニュアル類などドキュメントは可能な限り自動生成しています。解説が不足が生じている部分はありますが、網羅的にドキュメントが更新できることは大きなメリットです。翻訳テキストさえ準備すれば同じ形式のマニュアルを複数言語に対して準備することも難しくありません。
ただ一口に自動生成といってもいくつか段階を分けて対応が必要でした。コマンドの仕様をプログラム上のデータとして取り扱うこと、メッセージをソースコードから抽出すること、不足メッセージの検出とレポート、リリースノート向けの更新差分文章生成、リリースプロセスへの検査の組み込みなどをへて現在はある程度読めるマニュアル類が自動生成できるようになりました。
コマンド仕様がデータとして取り扱えるようになったことは嬉しい副作用もありました。コマンド仕様はリリースごとにJSON形式で格納しているのですが、このJSONを使ってたとえば、CSVファイルを引数にとるコマンドの一覧を抽出といった操作が簡単になりました。
% gzcat doc/generated/spec.json.gz | jq -r '.[] | select(.feeds | length > 0) | .path'
file dispatch local
file import batch url
group batch delete
member clear externalid
member delete
member detach
member invite
member quota update
member replication
member update email
member update externalid
member update profile
team activity batch user
team device unlink
team filerequest clone
teamfolder batch archive
teamfolder batch permdelete
teamfolder batch replication
ログファイルや中間ファイルでディスクを圧迫しすぎないこと
デバッグ作業効率を向上させるため、ログ出力部分には比較的多くの時間を費やしました。一方で、大きなフォルダやチームに対する操作は実行時間も長くなりログサイズも無視できない大きさになりました。ときに100GBを超えることもあります。このため、最近のリリースではログをgzip圧縮の上分割して、一定容量を超えた場合に古いログは削除するような仕組みを取り入れています。
また、古いログから順番に削除すると起動時のパラメータなど重要なデータが失われる場合があるのでそれらのデータは別のログに出力するなどの工夫も必要でした。
ログの圧縮やローテーション処理の実装自体はさほど難易度がたかくありませんでしたが、Windows上での安定性を向上させることには多くの時間を費やしました。マルチスレッド環境下でのデッドロックが原因です。ミューテクスのデッドロックが発生したり、I/O処理待ちがやはりデッドロックするなどいくつかの問題がありました。再現頻度は1〜数時間に1度程度で修正と修正の確認はなかなかの難易度でした。
今もすべての条件に対して耐久性があるとは考えていませんが、確率の高いデッドロックは概ね解消していると考えています。なおデバッガ接続中に再現したデッドロックが発生箇所は概ね次のようなコードでした。
type LogWriter struct {
w io.Writer
m sync.Mutex
}
func (z *LogWriter) Write(data []byte) (n int, err error) {
z.m.Lock()
defer z.m.Unlock()
return z.w.Write(data)
}
確かに、w
に対するWrite()
自体が何らかOSからロックされているとデッドロックされる可能性があります。詳しく仕様の調査はしていませんが、おそらくWindows上ではI/Oロックがより厳密であるためでしょう。
ブラックボックステスト的にわかることは、PowerShellなどコンソール上で何かテキストを選択するとスクロールがロックされ標準出力が停止します。これに従い、プログラム側も標準出力を待っていますのでプログラムが一時停止したようになります。macOSやLinuxではこのようなロックは発生しません。また同様の課題が発生した際には詳しく調査してみたいと思っています。
メモリの消費を概ね数百MB程度に抑えること
外部ライブラリを使うとメモリ消費が予想よりも一気に膨れ上がるケースがあります。現状いくつか大きくメモリをつかうライブラリについては対処策を組み込んでいます。具体的な例をいくつか紹介していきます。
watermint toolboxではレポートファイル出力フォーマットにCSVやJSONの他にExcelなどが利用するxlsx形式ファイルがあります。
xlsx形式は使わずCSVだけでも良いのですが、日本語が含まれるCSV (UTF-8エンコーディング、BOMなし)ではExcelで読み込もうとすると、ExcelはBOMがないためエンコーディングを正しく認識できず文字化けしてしまいます。CSV側にBOMをつけても良いのですが、BOMなしを期待するプログラムでは使い勝手が悪くなってしまいます。このため、カジュアルに使っても文字化けの問題を起こさないためにxlsx形式での出力もサポートするようになりました。
さてxlsx形式はOffice Open XMLのうちの一つで今はISO/IEC 29500として標準化されています。xlsx形式のファイルはzip圧縮されたいくつかのファイルの集まりで、メインのデータが含まれるファイルはXML形式で作られています。
スプレッドシートをXMLで表現するということは、スプレッドシート全体を一度DOMなどXMLツリーとしてメモリ上に展開する必要があるということです。スプレッドシートの容量が大きくなると比例してメモリを利用します。 このため、レポートとしてXML形式で出力しようとするとレポート行数に従い比例的に秋メモリ容量を食い潰していくことになります。場合によってはこれが原因でOut of memoryエラーとなり処理が停止してしまします。対策として、xlsx形式レポートは一定行数を超えた場合には別ファイルに分割して出力するようにしました。
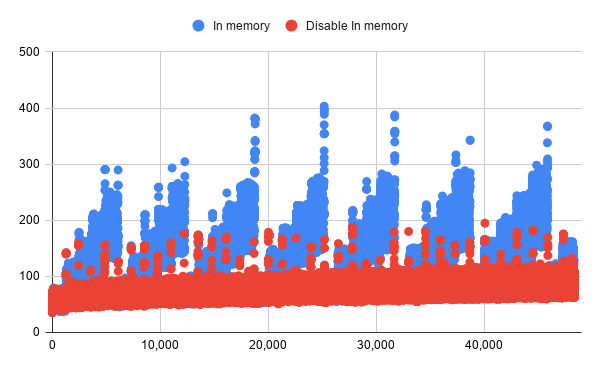
これ以外にもKVSとして利用しているBadgerもキャッシュのためにデータ件数に応じてメモリを利用するため幾度となくチューニングを繰り返しました。ここでも役に立ったのは、ログファイルからメモリの統計を取得する方法で、パラメータを変えた長時間の測定にも役に立ちました。上図は2つのパラメータ設定でのメモリ消費トレンドを示したものです。
耐障害性の向上と、実行速度の最適化
耐障害性向上と実行速度についてはまだ伸び代のある分野です。耐障害性について、API呼び出し時の自動リトライなどはすでに実装済みですが、フレームワーク全体としてのエラー処理については改善の余地があると考えています。
Go言語では複数の戻り値を使え、エラー処理のために最後の戻り値としてerror
を返すデザインが標準的です。watermint toolboxはビジネスロジックの中核であるコマンドと、それを支えるフレームワーク部分で構成されています。多くの場合、ビジネスロジック部分ではAPIから返されるエラーを処理しないためエラーが発生してもそのまま上位フレームワークへ返すよう実装しています。
func (z *List) Exec(c app_control.Control) error {
entries, err := sv_files.New(z.Peer.Context()).List(z.path)
if err != nil {
return err // 上位フレームワークへエラーを返す
}
// ... 後続の処理
return nil
}
ネットワークIOエラーなどはREST APIフレームワーク側で吸収していますし、これはこれで問題ないのですが、フォルダからファイル一覧を取得する際、ファイルがなければ別の処理をするといったビジネスロジック上必要なエラーなのか、認証エラーなどフレームワーク側で対応するエラーなのかまた判定ロジックを各所で書かなければならない点がいかがなものかと考えています。
最終的にまた現在の形に落ち着くかもしれませんが、フレームワークで吸収するものとビジネスロジック側で吸収するものを明示的に書き分けられるような仕組みを作るかもしれません。Try型のような実装の試みもあるようですし、エラーで返す場合とpanic()
を使うなど方法があるかもしれません。
実行速度の最適化についても改善の余地が大きく残っています。現在のコマンドはファイルのアップロードやフォルダの権限情報取得などある程度処理分散できるものについてはマルチスレッドで実行されるようプログラムしています。Go言語ではgoroutineやチャネルなど並列処理のための環境は整っているのであまり大きく悩まなくても実装できる点が気に入っています。
一方で、実行時間が数日以上などある程度長くなる場合にはエラーで停止した際に、また再開ポイントまでたどり着くための処理でオーバーヘッドが大きくなったり、中間ファイルが肥大化したり、進捗がわかりづらいといった課題もあります。このため、そろそろ永続化可能な非同期キューなどを用いた処理フレームワークを導入するなど検討が必要でしょう。
まとめ
リリースプロセスなどいくつか開発プロセス自体もツールの一部として取り込んだことにより、開発効率は4年前と比べれば格段に向上しています。一方で、Go言語やその取り巻くエコシステムのおかげで気軽に予想だにしなかった高機能な仕様を追加できるようになりました。開発当初はJavaやScala、Rubyのほうが経験値が高かったので苦労する部分もありましたがようやく大体の実装はGoでできるなという感覚が得られてきました。
また面白い実装方法を思いついたり、いいライブラリに出会えた際には紹介していきたいと思います。